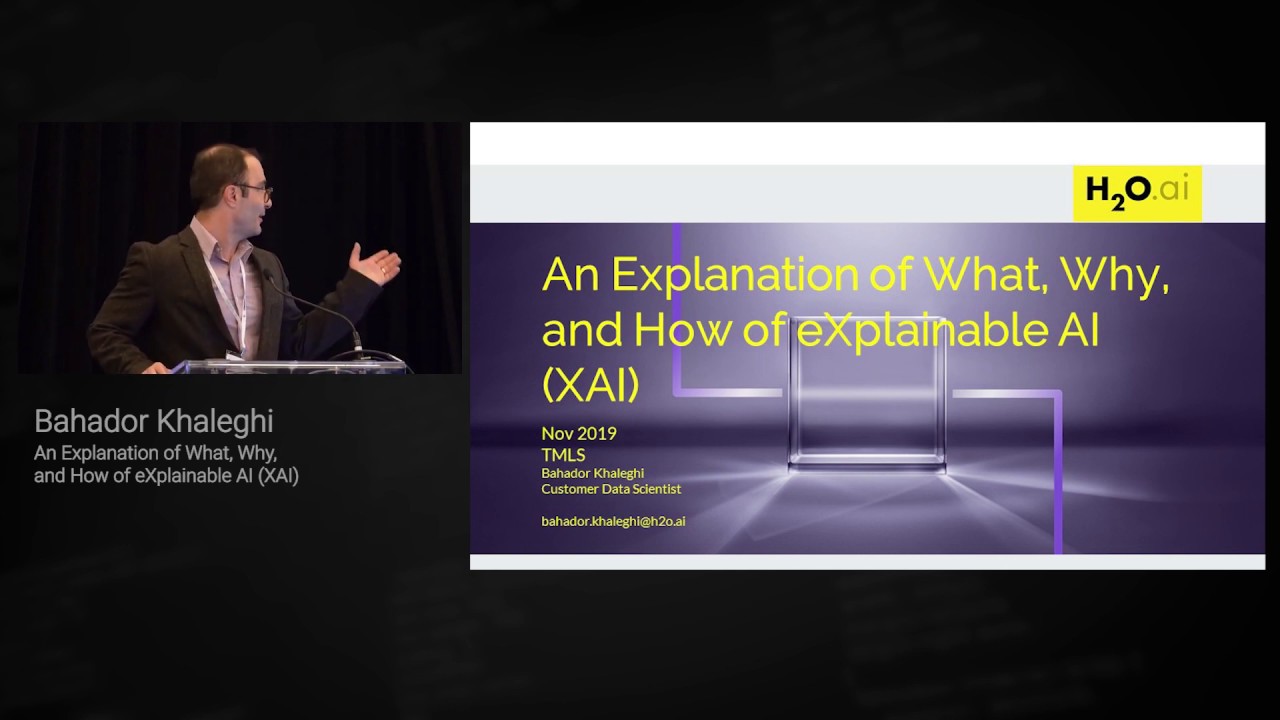
The video is hosted by
About the speaker:
Bahador Khaleghi is a Customer Data Scientist and Solution Engineer at H2O.ai. His unique technical background, which he has gained over the last thirteen years, is quite diverse entailing a wide range of disciplines including machine learning, statistical information fusion, and signal processing. Bahador obtained his PhD from CPAMI at the University of Waterloo. Over the last six years, he has actively contributed to industrial R&D projects in various domains including Telematics, mobile health, predictive maintenance, and customer analytics. Acting as the (former) technical lead of the explainability team at Element AI, he is currently focused on developing novel methodologies that enhance transparency, trustability, and accessibility of AI solutions."
About the talk:
"Modern AI systems are increasingly capable of tackling real-world problems. Yet the black box nature of some AI systems, giving results without a reason, is hindering the mass adoption of AI. According to an annual survey by PwC, the vast majority (82%) of CEOs agree that for AI-based decisions to be trusted, they must be explainable. As AI becomes an ever more integral part of our modern world, we need to understand why and how it makes predictions and decisions.
These questions of why and how are the subject of the field of Explainable AI, or XAI. Like AI itself, XAI isn’t a new domain of research, and recent advances in the theory and applications of AI have put new urgency behind efforts to explain it. In this talk we will present a technical overview of XAI.
The presentation will cover the there key questions of XAI: “What is it?”, “Why is it important?”, and “How can it be achieved?”.
The what of XAI part takes a deep dive into what it really means to explain AI models in terms of existing definitions, the importance of explanation users’ roles and given application, possible tradeoffs, and explanation studies beyond the AI community.
In the why of XAI part, we explore some of the most important drivers of XAI research such as establishing trust, regulatory compliance, detecting bias, AI model generalization and debug.
Finally, in the how of XAI part we discuss how explainability principles can be applied before, during, and after the modelling stage of AI solution development. In particular, we introduce a novel taxonomy of post-modelling explainability methods, which we then leverage to explore the vast XAI literature work."
0 Comments